The Revolution in AI technology: from Gpus to dedicated AI chips
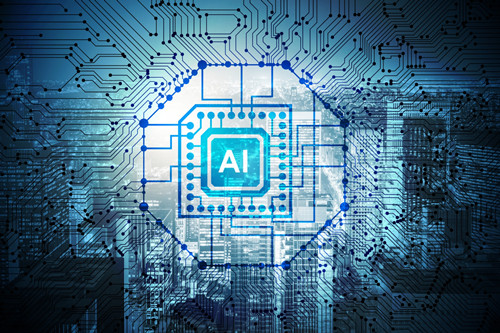
The big change from Gpus to dedicated AI chips. The development of AI technology is like riding a rocket, changing our life, work, and even the way we think about problems. AI can have today's development, thanks to many technological advances, especially changes in hardware. From the beginning of the general graphics processing unit (GPU), and then to the latter dedicated AI chip, the continuous innovation and upgrading of this hardware has laid a solid foundation for the development of AI.
At the beginning of the development of AI technology, Gpus were widely familiar. Because it has a lot of parallel computing power. The GPU was originally designed for graphics rendering, but those engineers were smart enough to quickly discover that it could greatly speed up large-scale data processing and complex algorithms. Compared with the traditional CPU (central processing unit), the CPU has fewer cores and low parallel processing capacity when processing specific computing tasks, but the GPU is not the same, it can handle thousands of data threads at the same time, especially suitable for applications in the field of big data such as deep learning.
With the increasing popularity of the 26WB35SN-UWSB1 deep learning algorithm, the GPU has become a good helper for training neural networks. Companies like NVIDIA are also starting to optimize GPU products specifically for deep learning, with a series of GPU architectures optimized for AI computing, like CUDA and Tensor Core. These technologies have greatly improved the speed and efficiency of AI model training, which used to take weeks to train a complex model, but now can be completed in a few hours, which can pave the way for the wide application of AI.
And then there's the rise of the AI chip. With the continuous development of AI technology, especially the increasing number of large-scale applications, the performance of GPU alone can not meet the increasing computing needs. In this case, dedicated AI chips appear. This dedicated AI chip is hardware designed for specific AI computing tasks, and its architecture is completely different from that of traditional cpus and Gpus. The emergence of AI chips like ASics (application-specific integrated circuits) and FPgas (field Programmable gate arrays) marked a new phase in AI hardware.
Asics are chips that are highly optimized for specific applications. For example, Google's TPU (Tensor Processing Unit) is a particularly efficient ASIC whose structure is specifically designed to accelerate deep learning. This TPU is very powerful when performing matrix operations, so when processing many neural network operations, the performance is better than the GPU.
In FPGA, it is programmable, developers can adjust at any time according to their needs. It is efficient and flexible when dealing with specific algorithms, but the performance may be slightly lower than ASIC. But in the development phase or in the test phase, because of its flexibility, the advantage is still quite large. Many companies are willing to use FPgas to quickly iterate and prototype AI models.
These AI chips are getting more and more diverse. With the increasing number of application scenarios of AI technology, a variety of dedicated AI chips have also emerged. Different AI tasks require different data processing capabilities. For example, natural language processing, image recognition, sound processing, these fields have their own special needs, so those chip manufacturers have begun to introduce a variety of AI chip solutions.
In recent years, the development of new energy vehicles and driverless industries has been called a boom, and the demand for intelligent hardware for AI chips has been rising. In order to be able to process in real time, but also low latency, these fields have higher performance requirements for AI chips, which has also promoted the development of a series of dedicated AI chips.
Looking at the huge computing needs of data centers, many chip research and development companies have begun to focus on high-efficiency and low-power AI chips. For example, some new neural network processors (Npus) have found a balance between performance and power consumption, becoming an important part of data center and edge computing. With these chips, enterprises can not only greatly reduce operating costs, but also improve the quality and response speed of AI services.
In addition to the progress of dedicated hardware, the rise of open source hardware has also added momentum to the development of AI technology. Many developers and researchers realize their AI applications through open source hardware platforms, which lowers the threshold of AI technology, and makes AI technology more popular and develops faster. With open source hardware, developers can more easily test and optimize their own algorithms, allowing AI models to iterate and innovate faster.
Open source hardware projects like the Raspberry Pi and Arduino have become important tools for learning and practicing AI technologies. They can not only support the operation of machine learning frameworks, but also interact with real-world environments through a number of sensors and peripherals, helping developers make smarter applications.
With the continuous progress of AI technology, the future AI chips may develop in the direction of higher integration and more intelligence. Based on the exploration of emerging technologies such as quantum computing and light computing, future AI chips may lead to another major change in the field of computing.
The rise of multimodal AI systems will also allow AI chips to move toward more complex computing needs. This system can process many types of data, such as images, text, audio, etc., which can promote AI in smart home, smart medical, robotics and other fields more widely used. To meet these needs, the architecture and design of AI chips will need to be more flexible and diverse to cope with the complex computing tasks of the future.
This development process from Gpus to dedicated AI chips reflects the rapid changes and innovations in AI hardware technology. Whether it is for individual users or enterprise applications, a variety of AI chips have brought unlimited possibilities to the future intelligent society. With the deepening of research and continuous breakthroughs in technology, the development of AI hardware will continue to promote artificial intelligence to a broader world.
The Products You May Be Interested In
![]() |
CAR2548FPBC-Z01A | AC/DC CONVERTER 48V 2500W | 5490 More on Order |
![]() |
PNVT003A0X43-SRZ | MODULE DC DC CONVERTER | 7308 More on Order |
![]() |
MDT040A0X3-ABSRPHZ | DC DC CONVERTER | 4734 More on Order |
![]() |
TJX120A0X3PZ | DC DC CONVERTER | 7344 More on Order |
![]() |
AXH010A0Y3 | DC DC CONVERTER 1.8V 18W | 5796 More on Order |
![]() |
APTH020A0X43-SRZ | DC DC CONVERTER 0.6-3.63V 73W | 8226 More on Order |
![]() |
SW003A5F94Z | DC DC CONVERTER 3.3V 12W | 3420 More on Order |
![]() |
QRW025A0A6Z | DC DC CONVERTER 5V 125W | 3690 More on Order |
![]() |
QRW025A0A1-H | DC DC CONVERTER 5V 125W | 2466 More on Order |
![]() |
MW005AJ | DC DC CONVERTER +/-5V 5W | 8172 More on Order |
![]() |
JW060ABK | DC DC CONVERTER 5V +/-12V 60W | 6822 More on Order |
![]() |
JC050C1 | DC DC CONVERTER 15V 50W | 6786 More on Order |
![]() |
JAW050A | DC DC CONVERTER 5V 50W | 2790 More on Order |
![]() |
HW010A0F | DC DC CONVERTER 3.3V 33W | 8334 More on Order |
![]() |
CC025ACL-M | DC DC CONVERTER 5V +/-15V 25W | 6138 More on Order |
![]() |
ATH030A0X3 | DC DC CONVERTER 0.8-3.6V 108W | 4644 More on Order |
![]() |
AXH005A0X | DC DC CONVERTER 0.8-3.6V 18W | 4716 More on Order |
![]() |
QBDW033A0B41-PHZ | DC DC CONVERTER 12V 400W | 5382 More on Order |
![]() |
JNCW016A0R41Z | DC DC CONVERTER 28V 448W | 2160 More on Order |
![]() |
ERCW003A6R641-HZ | DC DC CONVERTER 28V | 4320 More on Order |
![]() |
MVT040A0X43-SRPHZ | DC DC CONVERTER 0.45-2V 80W | 8136 More on Order |
![]() |
KHHD002A5B41-SRZ | DC DC CONVERTER 12V 30W | 6174 More on Order |
![]() |
SHHD005A0F41-SRZ | DC DC CONVERTER 3.3V 15W | 5526 More on Order |
![]() |
SHHD001A3B41Z | DC DC CONVERTER 12V 15W | 15600 More on Order |